Introduction
Online job information artificial intelligence (AI) services, called human resource technology (HR Tech), includes concepts such as web-based, online-based, digital, and smart. It is leading to new changes in job search and job search methods. Suppose you look at Saramin (http://www.saramin.co.kr), a site that introduces AI technology among online job information platforms in Korea. In that case, it is possible to search for personalized job information through an algorithm that continuously learns platform users’ activities.
Although many companies have applied AI to online job information platforms, empirical studies on AI service quality, preference, satisfaction, and continuous usage intention are still insufficient (van Esch, Black, & Ferolie, 2019). The purpose of this study is to clarify the continuous usage intention of AI products and services. To that end, this study tries to define the AI quality and preference on the online job information platform and investigates AI’s effect on continuous usage intention.
Theoretical Background
Online recruitment is being used to hire talented people efficiently to achieve an organizational competitive advantage in a rapidly changing global business environment (Son, Lee, & Chang, 2019). In the traditional online job search process, job seekers search for potential applying companies through search tools or referral companies on the job search online platform and fill in the information (personal details, resumes, qualifications, etc.) required by the company. On the other hand, in the AI-based job matching process, job seekers input their personal information before a job search. The AI learns their information in the online job information platform and recommends a potential job to the job seekers, as shown in Figure 1.
Quality related to information technology (IT) is redefined in various forms according to the context of research, as the boundaries between systems and services, information and services, and systems and information are overlapped. Although AI quality has not been clearly defined until recently, AI represents a computer technology level for clearly classifying and analyzing objects through learning, reasoning, and recognizing using data (Najafabadi et al., 2015; Stone, Neely, & Lengnick-Hall, 2018; Syam & Sharma, 2018). In other words, AI quality can be determined by how accurately the computer has learned a large amount of good quality data, and this machine learning is related to big data analytics (Chen, Chiang, & Storey, 2012). Big data analytics encompasses various data mining techniques, including artificial neural networks and optimization models. It includes quality factors related to descriptive, predictive, and prescriptive analysis according to the purpose of use.
The preference for AI can be understood by focusing on the following contents. Preference means superiority, indicating that something previously or more has been perceived by an individual (Muthitcharoen, Palvia, & Grover, 2011), resulting from previous usage evaluations and expected outcomes (Lee & Koubek, 2010). Preference by previous usage evaluation means taking into account the attributes of the product perceived by prior experience. Preference-based on expected performance indicates preference expressed by the evaluation information, preferring superiority when presenting a system or service with superior performance by objective data or recommendation. Preference is based on subjective judgment. It occurs in the case of previous repetitive decision-making behavior and the selection of new ones. It also appears to determine priorities among various attributes possessed by a specific object (Bettman & Zins, 1977).
The previous study of preference using contradiction theory may show that products’ familiarity may vary depending on enhanced functions or unique functions (Zhou & Nakamoto, 2007). As the more people are positive with familiar products, they may feel the importance of the product’s unique features, and less friendly products tend to take risks, so they prefer products with enhanced functionality that are exclusive to the market.
This study adopts the technology preference model (TPM) and the user’s information processing theory (IPT) to investigate AI’s influence on continuous usage intention. Based on the TPM, it is assumed that human information processing can be structured similarly to computer information processing (Muthitcharoen et al., 2011). As shown in Figure 2, when a piece of particular information enters into a person, a person performs a series of processes and derives the result. In this process, people can be influenced by internal factors (individual’s feelings and values) and external factors (social influence and technology diffusion) regarding using IT (Yoon & Tong, 2009).
As shown in Figure 3, the research model includes information processing processes such as (1) evaluation in the process of searching for information, (2) preference in comparison with previous experience, (3) attitude factor (satisfaction), and (4) behavioral intention.
AI quality is defined as information analysis quality (IA quality) representing the evaluation of the analysis itself and information providing quality (IP quality) describing how to give the analyzed information. In other words, AI quality derives the objective information by analyzing the data through learning, reasoning, and recognition by the machine. Lee and Lee (2009) suggested that online product and service users’ perceived quality may positively affect preference through a priori experience. Based on previous studies, the relationship between AI quality and AI preference was hypothesized as shown in Hypothesis 1.
-
Hypothesis 1: AI quality in the online Job Information platform will have a positive effect on AI preference.
Satisfaction is defined as an emotional appraisal and the positive emotions that appear by owning or using a product or service (Cronin, Brady, & Hult, 2000). Kuo, Wu, and Deng (2009) emphasized the importance of content, personalized services, and system quality in the mobile environment. They pointed out that mobile service quality can affect customer satisfaction and behavioral intention with the user’s cognitive judgment. Lightner (2003) argued that the quality and quantity of information could positively affect satisfaction in the online environment. Based on previous studies, the relationship between AI quality and satisfaction was hypothesized as shown in Hypothesis 2.
-
Hypothesis 2: AI quality in the online job information platform will have a positive effect on satisfaction.
Kuo et al. (2009) pointed out that the online platform’s service quality can positively affect continuous usage intention. Tseng (2015) suggested that the cloud-based personalization service includes information quality, system quality, and service quality and positively affects continuous usage intention. Based on previous studies, the relationship between AI quality and continuous usage intention was hypothesized as shown in Hypothesis 3.
-
Hypothesis 3: AI quality in the online job information platform will positively affect continuous usage intention.
Preference is defined as subjective evaluations using certain products or services (Zhou & Nakamoto, 2007). In thesss evaluation process, preference is an explicit level of comparison that can directly assess alternatives, affecting the attitude, which is an implicit comparative level in assessing user perceptions. Muthitcharoen et al. (2011) found that the attribute of online service products, purchase costs, and relative risk can influence the preference at the explicit level from technology acceptance. And they suggested that the preference at the explicit level affects the attitude, which is the evaluation at the implicit level. Thus, the preference for online stores can have a positive effect on satisfaction. Based on previous studies, the relationship between AI preference and satisfaction was hypothesized as shown in Hypothesis 4.
-
Hypothesis 4: AI preference in the online job information platform will have a positive effect on satisfaction.
Xiao and Benbasat (2007) suggested that the online user’s preference for information such as product characteristics, importance, and ratings can positively affect behavioral intention. Lee and Koubek (2010) suggested that the previous usage preference can positively affect the continuous usage of websites through the empirical analysis of the relationship between the subjective evaluation of website user experience and actual use. Based on previous studies, the relationship between AI preference and continuous usage intention was hypothesized as shown in Hypothesis 5.
-
Hypothesis 5: AI preference in the online job information platform will positively affect continuous usage intention.
Olsen (2002) empirically verified that satisfaction positively influences continuous usage intention by analyzing various products from a traditional perspective. Zheng, Zhao, and Stylianou (2013) suggested that customer satisfaction on the online platform can affect the continuous usage intention. Based on previous studies, the relationship between satisfaction and continuous usage intention was hypothesized as shown in Hypothesis 6.
Methods
This study explores the continuous usage intention of job seekers who use the online job information platform to apply and investigate the structural relationship between AI quality, AI preference, and satisfaction. Innovativeness is defined as the degree to which new technologies are quickly accepted, and AI preference is defined as the degree of preference for AI tools compared to other search functions and methods. For AI quality, IA quality and IP quality are defined as the overall level of AI analysis and the level of information provided through AI, respectively. Satisfaction is defined as the degree of AI service’s overall satisfaction on an online platform, and continuous usage intention is defined as the degree to which you continue to use AI services on an online platform.
Table 1 shows the measurement items for each construct and related studies of the research model’s variables proposed in this study. Specifically, AI quality is a second-order variable including two sub-variables, IA quality and IP quality. Continuous usage intention is a dependent variable, and innovativeness is a control variable. This study used a 5-point Likert scale to measure each variable of the proposed research model.
Construct | Item | Reference | |
---|---|---|---|
Innovativeness | The degree to which you seek advice on new technologies | Parasuraman and Colby (2015) | |
The degree to which you first acquire new skills between your friends and colleagues | |||
The degree to which you use new high-tech products or services without the help of others | |||
The degree to which you grasp the latest technology trends in the field of interest | |||
AI quality | Information analysis quality | AI’s overall job matching performance | Lee and Lee (2009) |
AI’s effective job matching performance | |||
AI’s stable job matching performance | |||
AI’s objective job matching performance | |||
AI’s exact job matching performance | |||
Information processing quality | The degree to which AI offers personalized job information (deletion) | Lee, Strong, Kahn, and Wang (2002) | |
The degree to which AI offers convenient job information | |||
The degree to which AI offers diverse job information | |||
The degree to which AI offers plentiful job information | |||
The degree to which AI offers trustworthy job information | |||
AI preference | The degree to which you select AI functions rather than existing search functions |
Lee and Lee (2009)
Muthitcharoen et al. (2011) |
|
The degree to which you like AI functions rather than existing search functions | |||
The degree to which you prefer AI functions rather than existing search functions | |||
The degree of suitability of AI functions rather than existing search functions | |||
The degree of meaningfulness of AI functions rather than existing search functions (deletion) | |||
Satisfaction | AI service’s overall satisfaction | Cronin et al. (2000) | |
AI service’s satisfaction on the information providing way | |||
AI service’s satisfaction on the usage determination | |||
The degree to which you think that it is good to use AI service | |||
The degree to which you think that it is wise to use AI service | |||
Continuous usage intention | The degree to which you reuse AI service | Kim and Niehm (2009) | |
The degree to which you use AI service continuously | |||
The degree to which you recommend AI service to others | |||
The degree to which you intend to use AI, if possible |
This study’s respondent is job seekers searching for job information in the online job information platform. Out of 9,290 questionnaires distributed using the Google Survey, 227 copies were collected (2.4% response rate) in the largest of Korea’s online job search community from 1 to 30 May in 2019. In the case of an online survey, there may be a large number of faithless respondents. As a result of checking the questionnaire contents, 43 copies were found to be incomplete and excluded, and 184 responses were used for the final analysis.
The main characteristics of the respondents who participated in the survey were male, 57.6% (106 people), and female, 42.4% (78 people). 15.8% (29 people) in their 30s, no people in their 40s, and 0.5% (1 person) in their 50s. The respondents’ characteristics found that those who frequently use online job search information are mainly job seekers in their 20s.
Before the hypothesis test, this study conducted the validation and reliability of the measurement model using SPSS 20 and SmartPLS 2.0. This study is an exploratory level of research, and the concept of measurement should be clarified. Therefore, exploratory factor analysis was performed to confirm the factor loading of the measurement item. Also, since AI quality is a second-order construct, a separate validity test needs to be performed. For this purpose, a hierarchical component approach was used. After the first test was completed, Cronbach’s alpha and composite reliability (CR) were calculated to test the variables’ internal consistency. Then correlation coefficients between variables and square roots of average variance extracted (AVE) were computed and compared to test each variable’s convergent validity and discriminant validity.
In this study, SmartPLS 2.0 was used to test the hypothesis. SmartPLS 2.0 can comprehensively analyze the coefficient of determination (R2) for endogenous variables of the research model and hypothesis tests through structural model analysis’s path coefficient. Gender, age, and innovativeness were used as control variables.
Results
Table 2 shows the results of the exploratory factor analysis. The factor loading must satisfy .7 or more, and each factor must be accurately classified into the corresponding concept. As a result of the analysis, two items (qua6, pre5) were deleted because they were not classified as relevant. The remaining factors were accurately derived according to the six constructs proposed in this study. The control variable, innovativeness, also met the criteria of the factor loading value.
Table 3 shows the reliability test results and AVE values of the measurement model. Cronbach’s alpha values for all variables in the research model are from .855 to .971 , and the CR values are from .902 to .942, meaning that it satisfies all of the typical values of reliability of .7 or more. Therefore, it was concluded that there is no problem with the reliability of the measurement model. All of the AVE values were also .5 or higher. Thus the convergent validity of the measurement model has been confirmed.
The discriminant validity was tested by comparing the AVE square root value of each latent variable and the latent variables’ correlation coefficient value. To secure the measurement model’s discriminant validity, each latent variable’s AVE square root value must be greater than the value of the correlation coefficient between the latent variables. As shown in Table 4, it was confirmed that the AVE square root value of each latent variable displayed on the diagonal line was higher than the correlation coefficient value between the latent variables resulting from the discriminant validity test.
In the case of AI quality, the validity was tested through second-order confirmatory factor analysis. Specifically, it is possible to test the validity of a research model having a second-order construct by comparing the factor loading values of the first-order factor and the second-order factor. The results of the hierarchical component approach are presented in Table 5. As shown in the table, the factor loading values of the second-order factor of AI quality were higher than those of the first-order factor. Therefore, the research model, including the second-order factor model, was found to be valid.
First, the online job information platform’s AI quality significantly affected preference (β = .507, t = 6.420). Therefore, hypothesis 1 was accepted. Besides, AI quality significantly affected satisfaction (β = .332, t = 3.939), and hypothesis 2 was also accepted. However, the AI quality did not significantly affect continuous usage intention, so hypothesis 3 was rejected. Here, in the case of an online job information platform that includes AI, it was found that behavior intention could be reached when AI’s overall quality and satisfaction are satisfied.
Second, AI preference had a significant effect on satisfaction in the online job information platform (β = .500, t = 6.614), hypothesis 4 was accepted. Also, AI preference significantly affected continuous usage intention (β = .365, t = 3.699), and hypothesis 5 was also accepted. It was found that preferences arising from previous experiences could continue to influence satisfaction and behavioral intentions.
Finally, AI satisfaction in the online job information platform significantly affected continuous usage intention (β = .372, t = 3.109), hypothesis 6 was accepted. In previous studies, satisfaction is the variable that has the most significant influence on behavioral intentions, and the same results were confirmed on AI online job information platforms. The summary of the test results from hypothesis 1 to hypothesis 6, direct effects, and structural equation analysis results are shown in Figure 4 and Table 6.
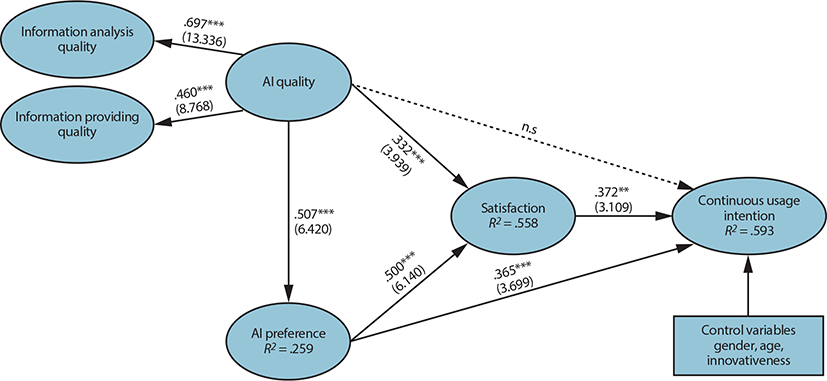
Discussion
The theoretical implications of this study are summarized as follows. First, AI quality was defined conceptually through the extensive literature review. As suggested in many previous studies, describing the quality of IT is the most critical process in understanding products and services. Delone and McLean (2003) argued that information quality, system quality, and service quality play a vital role in IT’s success. Zheng et al. (2013) discussed the importance of information quality and system quality in IT. Sahadev and Purani (2008) defined service quality from the viewpoint of IT. This study can have expanded the research on IT quality to the next level by defining AI quality academically. Second, previous studies showed a tendency to be limited to the IT acceptance model (Choi, 2019). However, this study suggested that the user’s objective evaluation and subjective evaluation of IT are considered simultaneously in accepting IT. Finally, the process of the intention to use IT was explained through the IPT. Like a computer, human decision-making determines behavioral intention through a series of information processing when certain information comes in. In other words, it was confirmed again that quality influences behavioral intentions through attitude, as in previous studies. In the case of preference, both direct and indirect influences on behavioral intentions were found. Rather than direct effects, higher indirect effects were appeared through the attitude of satisfaction. Through these results, it was again confirmed that attitude is essential in the acceptance of IT.
The practical implications of this study are as follows. First, satisfaction is an essential factor in developing AI products and services. The key to producing AI products and services is that technologies and services that are sufficiently satisfactory to AI users must be provided. As shown in this study results, to increase the satisfaction of AI, products, and services must be technologically advanced, and an environment that users can conveniently use must be created. Also, to enhance the user’s positive experience, it is necessary to continuously observe users of AI products and services and identify user needs changes. Second, this study presented the practical application method of AI in the online job information platform. It is crucial to provide job seekers with accurate job-seeking information among numerous job-seeking information. From a job seeker’s point of view, AI can help find the right talent among multiple applicants. It can have a positive effect on finding the most suitable company from a job seeker’s perspective. Therefore, it is expected that this study’s results contribute to developing both online job search and job information platforms in the early stages of introducing AI in the HR tech field.
This study has the following limitations. First, when measuring AI quality, it was performed only in terms of information provision. However, to precisely define and measure AI quality, it is necessary to measure AI quality, including the infrastructure, technology, and HRs. Second, the causal relationship between AI preference and satisfaction should be examined in more detail. In this study, AI quality was defined as the objective evaluation, and preference was defined as the subjective evaluation, and the study was conducted at the same level. However, some studies have shown that preferences and attitudes are related in similar areas. Therefore, it is necessary to analyze in depth what level to define and measure preferences and attitudes in future research. Third, risk factors of AI arising in analyzing users’ behavior patterns were not considered. AI presents the results of continuous learning by computers using data derived from human behavior patterns. In this process, personal information may be continuously leaked. Therefore, it is necessary to consider the risk factors of using AI in future studies. Finally, the limitations of generalization can be pointed out in this study. Since this study was conducted only on AI online job information platforms, it is necessary to consider various types of AI products and services such as AI assistants, chatbots, and robots for generalization of the study.
Nevertheless, this study offers considerable extensibility. This study’s research results can serve as primary data for understanding the essential factor for receiving continuous user selection when various AI products and services are currently being commercialized. It can also provide useful information for explaining AI’s characteristics that fusion with existing IT and has an essential meaning in expanding the existing IT area.
Conclusion
This study analyzed the continuous use intention of job seekers who use the online job search information platform applied with AI through the process of AI quality, preference, and satisfaction. The research scope is set for job seekers who are currently searching for job offer information in an online environment and who use the job information online platform. To this end, a survey was conducted for job seekers who currently use the online job search information platform. The empirical analysis results show that AI quality and preference positively affect satisfaction, significantly affecting continuous usage intention. AI quality, however, does not significantly affect continuous usage intention. This study suggests that the user’s objective evaluation and subjective evaluation of IT are considered simultaneously in accepting IT, based on statistically significant results. And this study presents the practical application method of AI in the online job information platform since it is crucial to provide job seekers with accurate and appropriate job-seeking information.