Introduction
The use of social media has experienced a significant increase with the advancement of the information and communications technology (ICT) field and the emergence of Web 2.0 (Thota, 2018). This rise has been further accelerated by societal changes, such as increased reliance on non-face-to-face communication, expanded digital infrastructure, and telecommuting. Social media sites, such as YouTube, Instagram, TikTok, and Facebook, have become significant communication tools and have revolutionized the way content is transmitted and consumed (Appel, Grewal, Hadi, & Stephen, 2020).
As consumers increasingly search for, acquire, and interact with products and brands online, sharing their experiences and opinions, businesses have recognized the importance of incorporating social media into their marketing strategies (Stephen, 2016). Social media marketing offers numerous opportunities for brand growth, serving as a valuable tool for building brand attitudes, reputations, customer relationships, and improving customer engagement (Bazi, Filieri, & Gorton, 2020; Holt, 2016; Ki, Cuevas, Chong, Lim, 2020; Sashi, 2012). Additionally, social media provides companies with a data-driven paradigm that allows them to establish close relationships with customers, easily access them online, and obtain information and opinions about products or brands through user-generated content (Adikari, Burnett, Sedera, de Silva, & Alahakoon, 2021; Dubbelink, Herrando, & Constantinides, 2021; Reveilhac & Blanchard, 2022; Sharma, Fadahunsi, Abbas, & Pathak, 2022).
To effectively convey a strong and clear message about a brand to customers who are constantly exposed to various social media marketing activities (SMMA), it is vital to have a specific understanding of and access to consumers who use social media platforms. As social media continues to expand as a critical marketing medium, multiple studies are being undertaken to study various aspects of SMM. Many of these studies apply structural equation modeling to analyze the relationships between variables. However, assumptions based on data from a single population can lead to misleading conclusions in multidimensional models. This is because there can be variations in perception, attitude, and behavior among different individuals (Rigdon, Ringle, & Sarstedt, 2010; Sarstedt & Ringle, 2010).
Previous studies have shown that consumers’ cognitive processes and acceptance of SMMA are influenced by various factors that shape their attitudes toward brands and their purchasing or word-of-mouth (WOM) behaviors (Blumler & Katz, 1974; Kim, Spiller, & Hettche, 2015; Stafford & Gonier, 2004). Therefore, it is vital to discover and understand customer heterogeneity and the influence of segments with similar characteristics on the level of awareness and acceptance of SMM efforts. In this study, we apply finite mixture partial least squares (FIMIX-PLS) to uncover unobserved heterogeneity and explain group-specific differences in the data resulting from this heterogeneity. FIMIX-PLS is a method that separates the entire dataset by identifying unobserved heterogeneity and takes into account the heterogeneity of the data by simultaneously estimating the path coefficients (Hahn, Johnson, Herrmann, & Huber, 2002; Sarstedt, 2008a).
This study aims to address the limitations of previous research on SMM operations, which often assume a homogeneous population and estimate models based on that assumption. The study aims to provide comprehensive insights into customers’ use of social media and to develop and execute more effective SMM strategies. The specific aims of this study are as follows:
-
Develop a comprehensive framework for SMMA that provides for data heterogeneity in customers’ acceptance.
-
Apply segmentation techniques to identify different types of social marketing consumers and prioritize targeted marketing strategies and measures.
By examining the relationship between SMMA, brand equity (brand image, brand awareness), brand attitude, and behavioral intentions (purchase intention, e-word of mouth intention), this study aims to segment the data into groups with heterogeneous characteristics and understand the consumer characteristics of each segment. The conclusions of this study will provide practical guidance for effectively implementing SMM strategies in the future.
Theoretical Framework
The theoretical framework employed in this study is the Stimulus-Organism-Response (SOR) model, originally proposed by Mehrabian and Russell (1974). This model posits that an individual’s emotional state is influenced by various stimuli, which in turn impact their consumption behavior. The SOR model demonstrates how external cues can trigger specific responses by stimulating an individual’s internal cognition and affective processes (Mehrabian & Russell, 1974). Drawing on the theoretical foundations of the SOR paradigm, this study has developed a research model, depicted in Figure 1, to investigate the diverse behavioral responses, such as purchase intention and WOM intention, influenced by cognitive and emotional internal states, collectively referred to as brand equity.
To examine the impact of SMMA on brand equity (brand image, brand awareness), brand attitude, and behavioral intention (word intention and purchase intention), the research model is developed based on existing literature.
The strategic use of social media in marketing plays a crucial role in establishing personal relationships with customers. Social media marketing (SMM) involves disseminating brand-related information through platforms such as Facebook, Instagram, YouTube, and Twitter to engage with various stakeholders (Albanna, Alalwan, & Al-Emran, 2022; Carlson, Rahman, Rahman, Wyllie, & Voola, 2021). This transition to social media has changed the way brand content is distributed and consumed (Appel et al., 2020). Social media marketing activities (SMMA) are a subset of online marketing that complement traditional promotional methods (Yadav & Rahman, 2018) and facilitating active interaction between companies and consumers, enhancing relationship communication. SMMA provides an opportunity to enhance brand value through the exchange of information and ideas about brands (Yadav & Rahman, 2017).
From an individual perspective, SMMA are highly beneficial for companies seeking to create value for their brands. They enable more specific identification of consumer feedback through real-time information provision and communication (Felix, Rauschnabel, & Hinsch, 2017; Zollo, Filieri, Rialti, & Yoon, 2020). Consequently, SMMA provides consumers with valuable content that enables more accurate predictions of purchasing behavior, increases brand awareness, retains existing customers, attracts new customers, and fosters a positive attitude towards the brand (Akar & Topçu, 2011; Jin, 2012).
These activities encompass elements such as interaction, fashionability, entertainment, and customization (Godey et al., 2016; Kim & Ko, 2012; Yadav & Rahman, 2017). Numerous studies have demonstrated the positive impact of SMMA on brand equity (Algharabat, Rana, Alalwan, Baabdullah, & Gupta, 2020; Schivinski, Muntinga, Pontes, & Lukasik, 2021; Seo & Park, 2018). For instance, Algharabat et al. (2020) found that consumers’ participation in brand-related activities on social media enhances brand awareness and brand association. Similarly, Seo and Park (2018) reported a positive effect of SMMA on brand awareness. Additionally, Schivinski et al. (2021) revealed that social media content positively impacts brand awareness. Laroche, Habibi, & Richard (2013) suggested that SMMA enhances the connection between consumers and brands by instilling positive brand awareness. In a study on luxury brand consumers, Godey et al. (2016) found a positive correlation between SMMA and brand image.
Based on these previous studies, the following hypotheses have been formulated.
-
Hypothesis 1: Social media marketing activities a) entertainment (ENT), b) interaction (INT), c) customization (CUS), d) trend (TRE), and e) information (INF) will have a positive effect on brand awareness (BAW).
-
Hypothesis 2: Social media marketing activities a) entertainment (ENT), b) interaction (INT), c) customization (CUS), d) trend (TRE), and e) information (INF) will have a positive effect on the brand image (BIM).
Brand awareness and brand image, which are components of brand equity, influence the formation of consumers’ attitudes. Recognized brands contribute to the development of positive attitudes toward those brands (Aaker, 1991). Martínez and Nishiyama (2019) described brand equity as comprising brand awareness, brand image, and brand loyalty, stating that brand image positively influences brand attitude. Alam and Khan (2019) examined the impact of social media content on repurchase intention, focusing on brand awareness and brand image, and found that brand image positively affects brand attitude. Additionally, customers’ perception of SMMA enhances their perception of the brand. The level of interaction between consumers and brands on social media is also positively associated with their attitude (Akar & Topçu, 2011; Pace, Balboni, & Gistri, 2017).
Based on these previous studies, the following hypotheses have been formulated.
Brand attitude refers to customers’ responses and preferences towards a brand and plays a crucial role in predicting their reactions to marketing activities. Brand attitudes are significant as they predict customer purchasing decisions (Chaudhuri, 1999). These attitudes are formed through brand recognition and evaluation, which subsequently influence behavioral intentions (Chu & Chen, 2019). According to the purchase intention theory, based on the theory of reasoned action and the theory of planned behavior, consumer attitudes directly influence behavioral intentions, which then affect purchasing behavior (Chang, Yu, & Lu, 2015). Gautam and Sharma (2017) argue that SMMA strengthen the relationship between companies and consumers, leading to a positive attitude towards brands and forming purchase intentions. Chiou and Cheng (2003) found a correlation between attitudes toward brands and online WOM. Furthermore, the more customers perceive SMMA as relevant, the more favorable their attitude towards the brand. Research also shows a positive relationship between consumer-brand interaction on social media and brand attitude (Akar & Topçu, 2011; Pace et al., 2017).
Based on these previous studies, the following hypotheses have been formulated.
Previous studies have shown that consumers are influenced by various factors when forming attitudes toward a brand through SMMA, which in tun affect their purchasing behavior and WOM. Consumers have been shown to differ in their level of awareness and acceptance of SMMA based on content type (Kim et al., 2015) and motivation (Stafford & Gonier, 2004).
Based on these previous studies, the following hypotheses have been formulated.
Methods
Participants in this study were individuals aged 20 and above with prior experience in SMM. A non-probabilistic convenience sampling method was employed to select a sample. Screening questions were used before conducting the survey to ensure the suitability of respondents. Consequently, only individuals with SMM experience participated in the survey. The survey was administered online, and a total of 521 completed surveys were included in the final analysis. Responses deemed untruthful or outliers were excluded from the analysis.
All model constructs were measured reflectively using multi-item questionnaires on a five-point Likert scale, ranging from 1 (“Not at all”) to 5 (“Very much”). Each item was adopted from previous literature on SMM and brand.
Specifically, entertainment (ENT) was measured using scales Godey et al. (2016), Noguti and Waller (2020). Interaction (INT) used scales by Jakic, Wagner, and Meyer (2017) and Yadav and Rahman (2017). Trendiness (TRE) was based on Ebrahim (2020). Customization (CUS) derived from Kim and Ko (2012) and Shanahan, Tran, and Taylor (2019). The measure for informativity (INF) utilized the item scale developed by Yadav and Rahman (2017). Brand image (BIM) and brand awareness (BAW) were measured using scales adapted from Godey et al. (2016), Sean Hyun and Kim (2011), and Kim and Ko (2012). WOM used scales by Godey et al. (2016) and Kim and Ko (2012). Purchase (PU) was based on Aaker (1997).
Although these item scales have been used in previous studies on SMM and brand, some modifications were necessary to account for differences in linguistic usage. After translating the scales from English to Korean, a pretest was conducted to ensure clarity and understandability.
The data collected for this study was analyzed using SPSS 25.0 (IBM, Chicago, IL, USA) and SmartPLS 3.0 software (SmartPLS, Bönningstedt, Germany). Initially, the data was screened, and descriptive statistical analysis was conducted using SPSS 25.0. Before conducting the Partial Least Squares-Structural Equation Modeling (PLS-SEM) analysis, the model was evaluated using a two-step analysis approach. The first step involved assessing the reliability and validity of the measurement model. The second step involved evaluating the structural model to test the hypotheses (Henseler, Ringle, & Sinkovics 2009). Both evaluations were performed using SmartPLS 3.0 software.
Next, path coefficient estimation and statistical significance of the model were tested using PLS-SEM. PLS-SEM is a prediction-driven approach that is suitable for estimating complex models and requires minimal assumptions about variable distribution, making it an appropriate analysis method for this study (Hair, Ringle, & Sarstedt, 2011; Rigdon, 2016). Additionally, FIMIX-PLS analysis was conducted to determine the heterogeneity of the structural model. FIMIX-PLS combines the Finite Mixing Model (FMM) with PLS-PM, using Ordinary Least Squares (OLS) and Maximum Likelihood Estimation (MLE) to estimate the path coefficients and identify unobserved heterogeneity, respectively (Hahn et al., 2002). This method allows simultaneous estimation of path coefficients while considering data heterogeneity, thus avoiding bias from individual model estimation (Sarstedt, 2008a). It is particularly useful in social sciences for explaining variations among different groups based on identified heterogeneity (Sarstedt & Ringle, 2010).
The results obtained from FIMIX-PLS were used to derive segment-specific characteristics influencing SMMA, providing strategic management insights.
Results
Among the respondents, 254 were men (48.8%) and 267 were women (51.2%), indicating a relatively even distribution. Most participants belonged to the age groups of 20–29 (27.4%), 30–39 (29.8%), and 40–49 (26.3%). Regarding educational levels, 43 participants were high school graduates (8.3%), 58 were junior college students/graduates (11.1%), 345 were students/graduates from four-year universities (66.2%), and 75 were students from graduate schools or higher (14.4%). In terms of average monthly income, 60.1% of respondents earned more than 2 million won. The survey on frequently used social media platforms (multiple answers allowed) showed that 281 people (53.9%) used Facebook, 384 (73.7%) used Instagram, 130 (25.0%) used Twitter, 387 (74.2%) used YouTube, and 189 (36.3%) used blogs.
The evaluation of the measurement model includes assessing internal consistency reliability, convergent validity, and discriminant validity. The results are summarized in Table 1.
Internal consistency reliability was assessed using Cronbach’s alpha (0.719 to 0.802), rho_A (0.731 to 0.805), and composition reliability (CR) (0.842 to 0.883), all of which exceeded the standard threshold, indicating high reliability.
Convergent validity was confirmed by actor loadings (0.729 to 0.863) and average variance extraction (AVE) (0.640 to 0.716), both exceeding the standard threshold.
Discriminant validity was tested using the Fornell and Larcker criterion (Fornell & Larcker, 1981), comparing the square root of AVE for each factor with the correlation coefficients between other factors. The square root of AVE for each factor was greater than its correlations with other factors, confirming discriminant validity. Detailed results are presented in Table 2.
Following the satisfactory evaluation of the measurement model, the structural model was assessed. This included examining the significance of path coefficients, variance inflation factor (VIF), coefficients of determination (R2), and predictive relevance (Q2).
Multicollinearity was checked using VIF, which ranged from 1.00 to 2.604, below the threshold of 5, confirming no multicollinearity issues. In PLS-SEM, the coefficient of determination (R2) is crucial for assessing the structural model. R2 values of 0.19, 0.33, and 0.67 indicate weak, moderate, and substantial explanatory power, respectively (Henseler et al., 2009). Thus, the model showed moderate explanatory power for BIM (R2 = .44), BAW (R2 = .57), WOM (R2 = .56), and PU (R2 = .56), and substantial explanatory power for brand attitude (BAT; R2 = .67).
Path coefficients and their significance were estimated using bootstrapping with 5,000 samples (Hair et al., 2011). The analysis revealed that all hypotheses, except H1d and H2d, were statistically significant at p < .01. Detailed results are presented in Table 3.
Endogenous constructs | Q 2 | R 2 | Effect level | |
---|---|---|---|---|
BAW | 0.351 | 0.672 | Substantial | |
BIM | 0.294 | 0.440 | Moderate | |
BAT | 0.447 | 0.571 | Moderate | |
WOM | 0.393 | 0.565 | Moderate | |
PU | 0.370 | 0.567 | Moderate |
Specifically, BAW was significantly influenced by ENT (β = .211, p < .001), INT (β = .274, p < .001), CUS (β = .193, p < .001), and INF (β = .157, p < .01), supporting H1a, H1b, H1c, and H1e. BIM was significantly influenced by ENT (β = .188, p < .01), INT (β = .287, p < .001), CUS (β = .281, p < .001), and INF (β = .105, p < .001), supporting H2a, H2b, H2c, and H2e. However, TRE did not significantly affect BIM and BAW. Both BAW and BIM significantly influenced on BAT, with BIM (β = .316, p < .001) having a greater effect than BAW (β = .570, p < .001), supporting H3 and H4. BAT significantly impacted WOM (β = .751, p < .001) and PU (β = .753, p < .001), supporting H5 and H6. Thus, the PLS-SEM analysis supported 12 hypotheses based on the aggregated data.
FIMIX-PLS is a latent class approach used to segment data by identifying unobserved heterogeneity (Hahn et al., 2002; Sarstedt, 2008b). This method follows a multi-level approach to segment modification and estimates segment-specific model paths (Hair, Sarstedt, Matthews, & Ringle, 2016). A major benefit of FIMIX-PLS is its ability to determine the optimal number of segments to retain in the data.
To determine the number of segments, various information criteria such as Akaike’s information criterion (AIC), Bayesian information criterion (BIC), and consistent Akaike’s information criterion (CAIC) are used. Since these values decrease as the number of segments increases, a smaller value generally indicates a better model fit. However, considering multiple criteria simultaneously often lead to a more optimal number of segments (Table 4).
The standardized entropy (EN) value indicates the reliability of each segment, with values closer to 1 suggesting clearer classification. Generally, the EN value should not be less than 0.5. Considering the complexity of the research model and the segment sizes shown in Table 5, the case of k = 3 segments is considered optimal.
Segment K | Segment 1 | Segment 2 | Segment 3 | Segment 4 | Segment 5 | Segment 6 |
---|---|---|---|---|---|---|
2 | 0.579 | 0.421 | ||||
3 | 0.546 | 0.284 | 0.170 | |||
4 | 0.432 | 0.271 | 0.168 | 0.129 | ||
5 | 0.251 | 0.227 | 0.180 | 0.176 | 0.166 | |
6 | 0.334 | 0.220 | 0.213 | 0.089 | 0.087 | 0.057 |
After determining the optimal number of segments, an evaluation of the segment-specific structural model derived from FIMIX-PLS is performed. Table 6 presents the overall data analysis results as well as the results for each segment. It is important to examine whether the unobserved heterogeneity in the clustering results into three segments is well accounted for by identifying between segments. This can be tested using multigroup analysis (MGA). The MGA results show that there are no significant differences in the path coefficients between segments, except for a few path coefficients.
In this study, the heterogeneity of consumers’ SMMA was confirmed using FIMIX-PLS. To further characterize segments separated by unobserved heterogeneity and explain different types of consumers, an Importance-performance map analysis (IPMA) was conducted. IPMA utilizes the average values of latent variables and path coefficient estimates to identify key independent variables impacting the final dependent variable (endogenous variable) and areas with relatively low performance levels.
Using brand attitude as the target dependent variable, the IPMA results are shown in Figures 2–4. These figures depict the four quadrants of the IPMA framework. In the first quadrant, there is a high level of importance and performance for the target dependent variable, which in this case is brand attitude. The second quadrant indicates a high level of importance for brand attitude but also suggests room for improvement, as the current performance level is low. The third quadrant reveals a low level of performance and importance for brand attitude. Lastly, the fourth quadrant demonstrates a high level of brand attitude performance but a low level of importance.
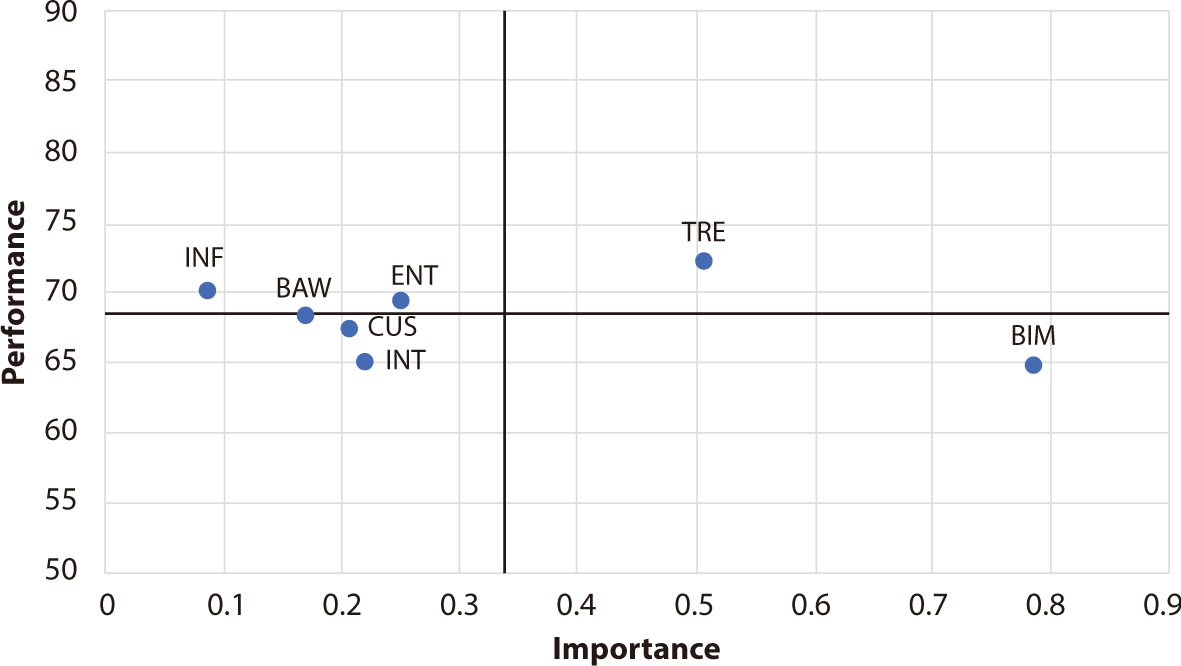
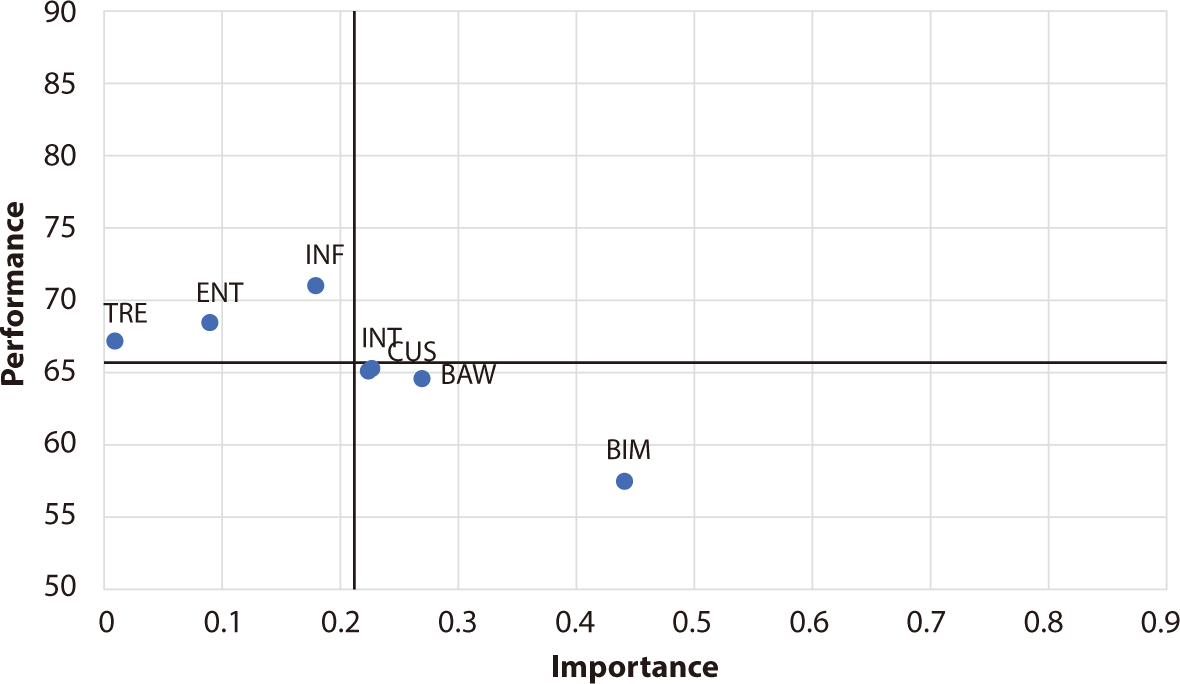
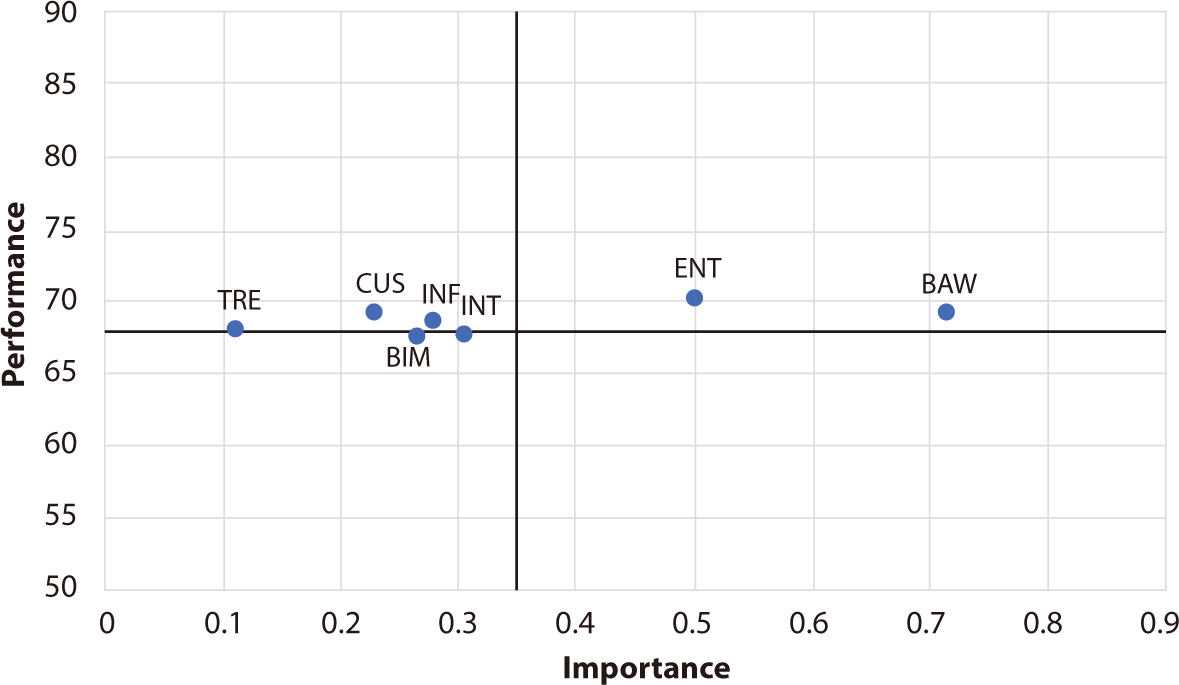
The IPMA analysis provides valuable insights into the relationship between importance and performance for various consumer segments in SMMA, helping to identify strategic areas for improvement.
Discussion
As the use of social media for customer interaction becomes more prevalent, effective management of social media has become increasingly important. Recognizing that companies’ approaches to reaching customers are evolving, it is crucial to acknowledge that customers now have greater access to companies, products, services, and brands, as well as more ways to evaluate them. Consequently, it is more efficient for companies to employ marketing strategies tailored to specific segments rather than treating all customers as a single group.
This study aims to achieve a multidimensional understanding of users in SMM by utilizing FIMIX-PLS to identify unobserved heterogeneity among different user groups. The results reveal that the user group for SMM can be divided into three segments. The characteristics of each group are examined based on the estimated path coefficient for each segment and the IPMA results.
First, the study aims to gain a comprehensive understanding of how SMMA influence brand equity (brand awareness and brand image), brand attitude, and behavioral intention (WOM and purchase intention). Among various SMMA, interaction factors have the greatest influence on brand awareness and brand image. This suggests that consumers attach significant importance to their interactions with brands. Previous research by Bilgin (2018) found that factors such as entertainment, interaction, and customization significantly impact brand recognition. Similarly, Zarei, Farjoo, & Bagheri Garabollagh (2022) and Seo and Park (2018) discovered that brand awareness is influenced by entertainment, interaction, customization, and trendiness. This study confirms that interaction has a consistent impact on brand awareness, and entertainment and customization are shared determinants of brand awareness.
Secondly, the study reveals that brand image positively impacts brand attitude, consistent with previous research findings (Akar & Topçu, 2011; Pace et al., 2017). These results indicate that emotional factors play a more significant role than cognitive factors in shaping consumers’ attitudes toward a brand. Therefore, companies should employ brand image strategies, such as consumer commitment strategies and customer value innovation strategies, to foster a positive attitude among customers.
Third, the study demonstrates that the trend factor in SMMA has a negative effect on brand image. While promoting a new product through trendiness positively impacts brand awareness, it can negatively affect brand image as consumers may perceive it as a promotional tactic. Seo and Park (2018) found that trendiness positively influences brand image, contrasting with the present study’s findings. This discrepancy may be due to the specific context of the Seo and Park study, where trendiness was associated with high pricing, aviation, and technology. This suggests that the impact of trendiness on brand image may vary depending on the product nature.
The study highlights the importance of identifying unobserved heterogeneity within a seemingly homogeneous population and segmenting the data accordingly. The findings align with prior research using FIMIX-PLS for segmentation (Haverila, Haverila, & McLaughlin, 2023; Joshi & Chawla, 2023; Yuan et al., 2021). These results indicate that FIMIX-PLS is an effective and suitable approach for analyzing SEM models, particularly when considering unobserved heterogeneity among customers.
Finally, by employing FIMIX-PLS to identify unobserved heterogeneity, the study divides the population into three segments and characterizes each segment as follows:
Segment 1: Comprising 223 individuals (42.8% of the total sample), this segment is primarily composed of individuals in their 40s (30.5%) and 20s (28.3%). Consumers in this group place significant importance on SMMA and brand image. Brand image is perceived as more influential in shaping brand attitude than brand awareness, suggesting that these consumers value the social and psychological aspects of a brand. This segment is labeled as a group that uses social media for self-expression.
Segment 2: Comprising 159 individuals (30.5% of the total sample), this segment values customization and interaction factors in SMMA, as well as brand image. Notably, 44.7% of this segment primarily uses Instagram, which facilitates easy interaction through direct messages and provides access to a wide range of personalized information. This segment is identified as a group that uses social media to gather diverse information about brands.
Segment 3: Comprising 139 individuals (26.7% of the total sample), this segment has a diverse age distribution and places significant importance on entertainment factors in SMMA. Unlike the other segments, customization and interaction are of low importance and performance. Additionally, 51.8% of this segment primarily uses YouTube, demonstrating a strong influence on brand attitudes towards brands frequently exposed through videos. This segment perceives other marketing activities as less important compared to entertainment elements, thus labeled as a group that uses social media for entertainment purposes.
Conclusion
Understanding the consumer segments is crucial for effective SMM. By delivering valuable content through social media platforms, companies can accurately predict customer behavior and cultivate loyal customers. Identifying consumer characteristics through segmentation is essential for developing customized marketing strategies. Effective communication in marketing relies on targeting the correct audience.
Brand recognition plays a significant role in capturing consumer interest by creating a positive perception of brands. Therefore, when promoting brands and their products, it is efficient to focus on improving brand image and awareness through SMM strategies. For instance, to increase awareness of brand A, creating engaging social media content and leveraging influencers can effectively enhance brand recognition.
Furthermore, consumer attitudes toward brands differ based on their level of engagement with social media. Hence, planning customized marketing strategies for each type of social media user is necessary. For users engaging in social media for self-expression, marketing efforts should incorporate trending elements. Users seeking information on social media require personalized marketing and timely communication. Lastly, users enjoying social media for entertainment purposes require marketing strategies with enjoyable and captivating elements.
For example, for brands associated with self-expression, marketing strategies should involve renowned influencers and incorporate recent popular elements like design and ambiance. Brands introducing new products or informing consumers about their offerings can utilize AI or big data to provide personalized information and enhance customer interaction through chatbots. Additionally, for brands aiming to reach a wide audience and generate positive brand attitudes, planning product placement marketing to frequently expose consumers to the brand can be effective.