Introduction
Metacognition is a higher-order cognition that allows people to be aware of and control their own cognitive processes involved in decision-making and learning (Cho & Linderman, 2019; Flavell, 1979; Kaberman & Dori, 2009). Briefly, metacognition can be described as cognition about cognition (Flavell, 1976). Studies have shown that this metacognitive awareness leads to better decision-making (Argyris, 1991; Berardi-Coletta, Buyer, Dominowski, & Rellinger, 1995; Swanson, 1990). Metacognition is thus considered a key concept in the problem-solving process (Celiker, 2015; Hartman, 1998).
In social science, metacognition is largely sub-conceptualized into metacognitive knowledge and metacognitive regulation (Baker & Brown, 1984; Schraw, Crippen, & Hartley, 2006). Specifically, metacognitive knowledge relates to individuals’ knowledge about their own cognitive processes, task, and strategies (Boyle, Rosen, & Forchelli, 2016; Flavell, 1979). Metacognitive regulation refers to planning, monitoring, and evaluating cognitive activities during learning and problem-solving tasks (Schraw, 1998). Field-specific studies on the conceptualization of metacognition are summarized in Table 1.
Study | Discipline | Contribution | Components |
---|---|---|---|
Schraw and Dennison (1994) | Educational psychology | Develop a 52-item inventory to measure adult’s metacognitive awareness. | Knowledge about cognition (declarative knowledge, procedural knowledge, conditional knowledge) Regulation of cognition (planning, information management, monitoring, debugging, evaluation) |
Mokhtari and Reichard (2002) | Educational psychology | Assess student’s metacognitive awareness of reading strategies. | Global reading strategies, problem-solving strategies, support reading strategies |
Haynie (2005) | Entrepreneurship | Explore the role of metacognitive awareness in entrepreneurial decision policies. | Goals and motivation, metacognitive experience, metacognitive knowledge, metacognitive monitoring, metacognitive control |
Van Dyne et al. (2012) | Social psychology | Conceptualize the metacognitive cultural intelligence (CQ). | Metacognitive planning, metacognitive awareness, metacognitive checking |
Cho and Linderman (2019) | Operations management | Explore the impact of managerial metacognition on process improvement practices. | Goal orientation, metacognitive knowledge, metacognitive experience, metacognitive strategy, monitoring |
Based on this conceptualization of metacognition, many studies have been conducted to explore how students’ metacognitive awareness affects their learning outcomes in various educational fields such as chemistry, biology, mathematics, and engineering (Aleven & Koedinger, 2002; Cook, Kennedy, & McGuire, 2013). There is broad agreement that students with higher metacognitive abilities are more adept at problem-solving and learning than those with lower metacognitive abilities (Flavell, Miller, & Miller, 2002; Swanson, 1990). Moreover, previous studies have demonstrated that students who have acquired metacognitive skills learn more effectively than those who have not (Cook et al., 2013; Kaberman & Dori, 2009; Zimmerman & Schunk, 2001).
Although metacognitive effects on the learning process have been studied in many other academic disciplines, there is little understanding of the relationship between students’ metacognitive awareness and learning effectiveness in business education. Furthermore, in the field of operations management, there has been no research to date on how teaching metacognitive learning skills affects students’ learning performance. Therefore, to fill this research gap, this study aims to answer the following research questions: (1) Is students’ metacognitive awareness related to their learning effectiveness in operations management education? (2) Can students improve their learning outcomes in operations management courses by training in metacognitive learning skills?
Methods
The participants in this study were 50 undergraduate students majoring in business administration at a state university in the United States. They were all taking a supply chain management (SCM) course in the same semester. One class with 23 students became the experimental group, and another class with 27 students became the control group. Both classes were based on the same curriculum and were taught by the same instructor. The study participants included 41 students aged 18–29 and 9 students aged 30 or older, of whom 15 were male, and 35 were female. Based on the guidelines of Caruana, Roman, Hernández-Sánchez, & Solli (2015), this study was designed as a longitudinal study, following the same participants over a semester. At the beginning of the semester, with their consent, a metacognitive awareness test was administered to all students, including both the experimental and control groups. On the other hand, only the experimental group received the metacognitive test results and instructions on interpreting the results, which took approximately 30 minutes. The SCM class had three course exams during the semester. After taking the exams, the experimental group was asked to perform an individual assignment to evaluate their exam results and develop future learning strategies from a metacognitive perspective. Details of the metacognitive awareness exercise on exam results are provided in Appendix 1.
Metacognition refers to a higher-order cognitive process of thinking that makes decisions (Flavell, 1979). Understanding which learning process is most effective for students can serve as a foundation for developing more effective metacognitive learning strategies. Hence, the experimental group was trained to evaluate their own learning process and develop learning strategies based on the results of the learning style assessment, which was originally developed by Robbins and Hunsaker (2011, pp. 17-21) based on the research of Kolb (1976, pp. 21-31). More information about this exercise can be found in Appendix 2.
A multi-item scale was developed to measure metacognitive awareness based on previous studies. The survey questions of metacognitive awareness consisted of items to measure metacognitive knowledge (i.e., declarative knowledge, procedural knowledge, conditional knowledge) and metacognitive regulation (i.e., planning, monitoring, evaluation, debugging, and information management) (Schraw & Dennison, 1994). The measurement items were adapted from the studies of Schraw and Dennison (1994) and Mokhtari and Reichard (2002). However, while the metacognitive awareness measure developed by Schraw and Dennison (1994) was intended for general adults, our study primarily focused on student learning, so the measurement scale was slightly adjusted to fit the purpose of this study. For each item, survey participants were asked to indicate the extent to which they agreed or disagreed with the statement using a 7-point Likert scale, ranging from (1) strongly disagree to (7) strongly agree. Further details on the metacognitive scales used in this study are presented in Table 2. Additionally, students’ learning effectiveness in operations management education was measured by the scores of three exams administered during the semester in the SCM course.
The reliability and validity of the measurement were tested. First, Cronbach’s alpha values for most variables, except declarative knowledge and monitoring variables, met the acceptable reliability level of .70 or higher (Cronbach, 1951; Nunnally & Bernstein, 1994), as shown in Table 2. Next, confirmatory factor analysis (CFA) was performed to assess the validity of the measurement. The ‘Metacognitive Awareness Scale for Adults’ by Schraw and Dennison (1994) originally consisted of 52 scale items. However, based on the test result of CFA, 21 items whose factor loadings did not reach the suggested threshold of .50 were deleted (Hair, Black, Babin, & Anderson, 2010, p. 686). As a result, the remaining 31 scale items were included in the final research model, and all items met the .50 criterion, ranging from .54 to .98. The details of all evaluation test results for the survey measures are presented in Table 2.
Results
To answer the first research question of this study, Is students’ metacognitive awareness related to their learning effectiveness in operations management education?, we conducted a structural equation modeling (SEM) test based on students’ survey data. The data samples used in this SEM test were collected from all participants in the experimental and control groups. Students’ metacognitive awareness was assessed with voluntary consent, and seven students in the control group did not participate, so the total sample size used in the SEM test was forty-three.
Figure 1 reports the test results from the SEM analysis using the IBM AMOS software. Figure 1 summarizes the results of the SEM test on the relationship between students’ metacognive knowledge, metacognitive regulation, and learning effectiveness in the SCM course. The results indicated that metacognitive knowledge was significantly related to metacognitive regulation (β = .918, t = 4.501, p < .001). Additionally, our research results showed that metacognitive regulation had a significantly positive impact on the learning effectiveness of students taking a SCM course (β = .446, t = 2.703, p = .007).
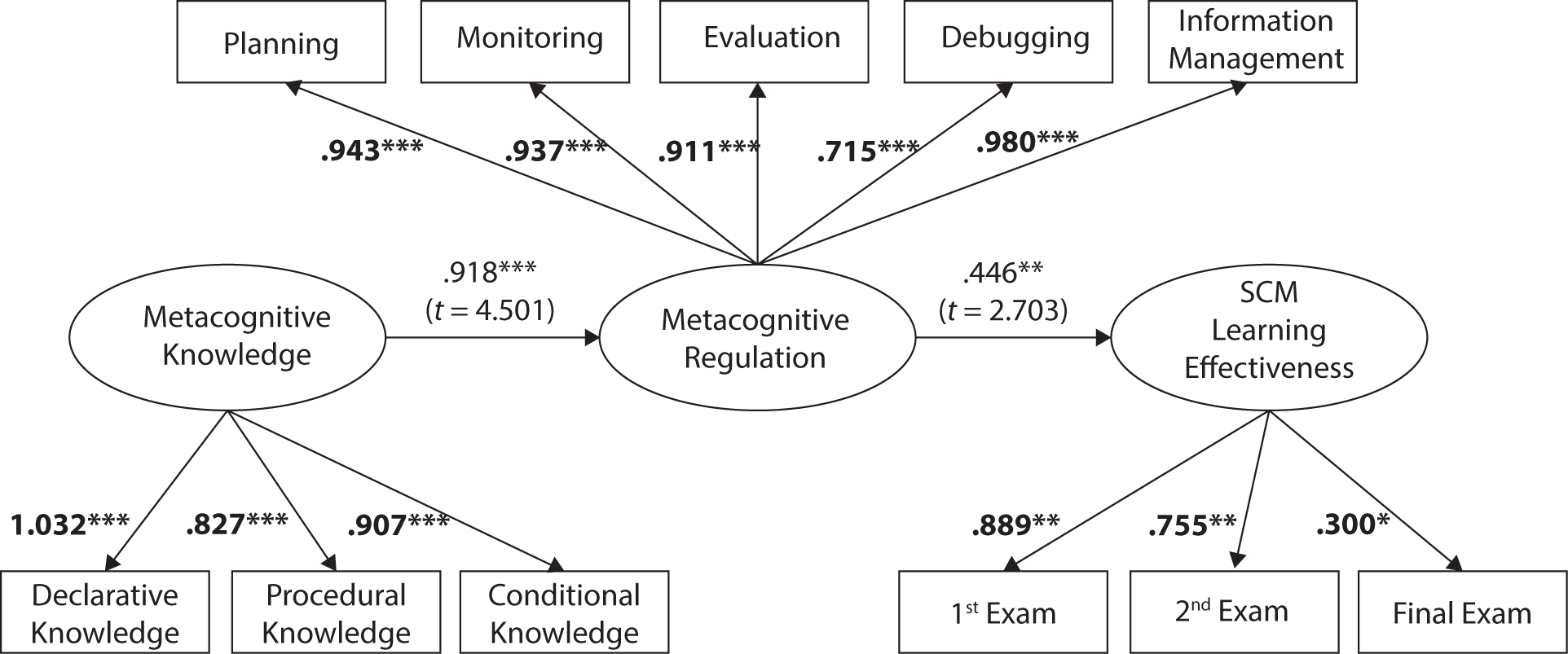
Analysis of variance (ANOVA) is often adopted for longitudinal analysis (Caruana et al., 2015), so we conducted ANOVA to examine our second research question, Can students improve their learning outcomes in operations management courses by training in metacognitive learning skills? We tracked changes in test scores for the experimental and control groups while the experimental group practiced metacognitive learning skills twice during the semester. Table 3 shows the change in mean scores of the experimental and control groups on three SCM tests administered during the semester. The ANOVA test results indicated no statistically significant difference between the mean scores of the two groups on the first SCM exam (p = .152), but on the final SCM exam, the mean scores of the experimental group (Mean = 25.91, n = 23) were significantly higher than those of the control group (Mean = 22.66, n = 27) (p = .010), as shown in Table 4.
Discussion
The results of this study have several practical and theoretical implications for business education. First, as mentioned earlier, although metacognition research has been conducted in various academic fields over the past 40 years to explore the role of metacognition in students’ learning processes, research in business education is still in its infancy. However, metacognitive learning skills are an essential quality for college students majoring in business administration, especially operations management. That’s because business education is fundamentally an academic discipline that deals with strategic decision-making related to business activities. In particular, the operations management perspective enables a more systematic and quantitative approach to these decision-making processes. Previous studies have shown that entrepreneurs with higher metacognitive awareness are more likely to seize early business opportunities and achieve entrepreneurial success (Cho & Jung, 2014; Haynie, 2005). Empirical studies have shown that managerial metacognition significantly impact the effectiveness of various process improvement practices such as total quality management (TQM), six sigma, and business process reengineering (Cho & Linderman, 2019). In light of these arguments, teaching metacognitive skills to business students should be considered an inevitable and urgent task of business education to help them become effective strategic decision-makers.
Second, the results of this study proved that metacognitive awareness was significantly related to students’ learning effectiveness in the SCM course. However, the SEM test results of this study showed that metacognitive knowledge, which is a component of metacognitive awareness, does not directly affect learning effectiveness and that students can achieve effective learning outcomes only when they have appropriate metacognitive regulation abilities. Thus, when developing a metacognitive curriculum for business education, greater emphasis should be placed on improving metacognitive regulation (i.e., the ability to manage one’s cognitive processes) rather than metacognitive knowledge (i.e., knowledge about one’s cognitive processes).
Last but not least, the results of this study presented that training in metacognitive learning skills positively affected students’ academic achievement. That is, the average final exam score of the experimental group who practiced metacognitive learning skills during a semester was significantly higher than that of the control group who did not practice metacognitive learning skills. However, there was no statistically significant difference between the experimental and control groups in the first exam. These results are similar to those of Cook et al. (2013), who studied the effects of metacognitive learning strategies on learning outcomes in chemistry courses. Consequently, the results of this study also provide empirical support for the metacognition theory that metacognitive ability can be improved with appropriate training, ultimately leading to better job performance (Nietfeld & Schraw, 2002; Schmidt & Ford, 2003).
Conclusion
This study explored the relationship between students’ metacognitive awareness and learning success in operations management education. The results of this study showed that (i) metacognition is significantly related to learning effectiveness in SCM courses, and (ii) metacognitive awareness can be improved through classroom training. These findings imply that the metacognitive lens is a more fundamental and practical framework for problem-solving processes in various business activities. With the AI-driven fourth industrial revolution, today’s managers face more complex and multidisciplinary challenges than ever. To respond to this business environment and maintain competitive advantage, managers must have metacognitive insights that act as a framework for continuously reflecting on existing business practices and discovering innovative alternatives. Accordingly, teaching metacognitive skills to business students is now seen as an inevitable and urgent new mission of business education to help them become effective decision-makers in a dynamic industry environment. Business schools can no longer function as catalysts and educational hubs of innovation unless they innovate their own curricula. Therefore, we hope this study will serve as a stepping stone for developing curricula that foster managerial metacognitive capabilities in future business education.